The Age of Information Over-Abundance
Humanity has come a long way throughout modern history. Innovation everywhere. Planes, trains, automobiles, rockets to the moon & beyond, microprocessors, the internet, social media, now virtual reality, artificial intelligence, and who knows what’s next. Exhilarating. Along the way as our technology has progressed, so too has the media by which we find out & communicate with each other about it.
With all this amazing innovation, we’ve solved away the vast majority of humanity's problems… in some places, almost to the point of excess (ie. food, entertainment, etc). Now, the days of scarcity are over, and new generations face a new dilemna: the problem of overabundance. Perhaps nowhere is this more true than in the realm of news & information.
Since the first newspapers were published back in the 1700’s, the amount of information published per unit time has increased exponentially — and shows no signs of slowing down. Call it the Moore’s Law of content. While the exact volume of news & online content created each year is difficult to estimate, we know that it’s big and growing at a rapid pace. According to Cisco, as of 2021 the amount of information transferred via the internet surpassed 5 zettabytes (or 4.8 trillion gigabytes) per year.
Lack of information will not be an issue for future generations.
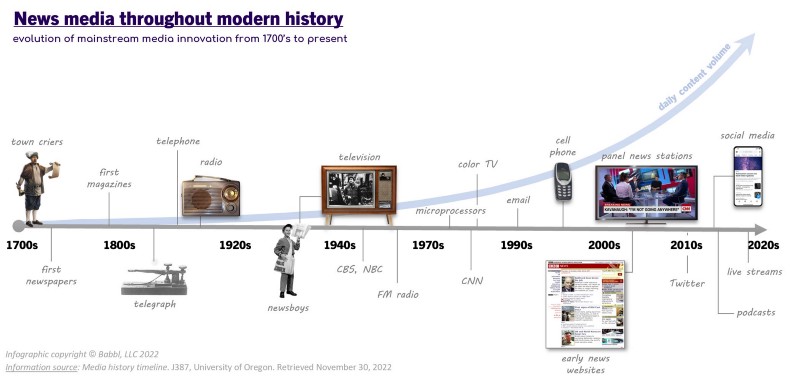
In our everyday lives, on our own personal journeys to parse the golden commodity of information we need to achieve our short- and long-term objectives, we are faced with a daily bombardment of ever-increasing news to pay attention to. In this golden age of data & content, if information is the ultimate asset, then our attention has become the ultimate currency. Perhaps unbeknownst to us, thousands of companies / millions of individuals are constantly competing to direct even a sliver of our fleeting attention toward their own content, with masses of clickbait & bots weaponized with the goal of keeping us on screen.
The Ultimate Currency: Attention
Perhaps nowhere is this currency of attention more apparent than in the realm of stock market investing. Imagine you are a stock market investor, building a portfolio to generate an income, buy a car, save for retirement, or send your kids to college — the American dream. As an independent investor or money manager, one of your most important activities is to parse the news so you can try & find which stocks could be worth buying or selling; at the very least, to find which stocks could be worthy of a deeper look.
Sure, you spend your money on these stocks with the hope of compounding that money into wealth; but perhaps more tangibly/directly, you’re spending your attention to acquire the information about which stock decisions will be correct over time. You want to know what Everyone thinks about a stock, to gauge the temperature of the mass mood. But you quickly realize: there’s thousands of sources and millions of posts / opinions / factoids you could be spending your precious attention on. More posts, articles, and comments per day than you could read in an entire year.
And to make things more complicated, the vast majority of these news sites (CNBC, Yahoo Finance, etc.) are free, only making money to keep the lights on by serving advertisements to their readers. In other words: the primary business objectives of most news sources is to write a high volume of clickbaity, low-value content to suck you in keep you on screen for as long as possible — with the ultimate goal of selling you advertisements. In the majority of cases, news providers are directly motivated to make finding actionable insight an inefficient and time-consuming process for their readers. It’s a complete misalignment of incentives.
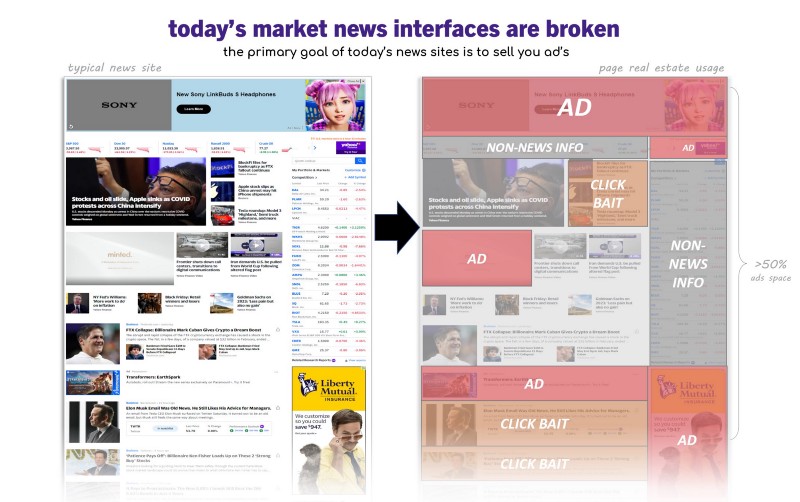
So what do you do? Most enterprising investors resort to one of two options: 1) either disregarding it all & living in blissful ignorance of the minute market-moving details, buying & selling on a whim or when their friends do, or 2) spending hours per week, doomscrolling through news & social media, hanging on for the right nugget of information, but mainly for fear of missing out on something important (FOMO).
This is the way the system is… for now. Doomscrolling, information overload, news fatigue. Stock market success remains a function of time & attention spent finding the right insights at the right time & avoiding the wrong ones — we haven’t been able to innovate our way out of that one yet. Time = money.
But… perhaps there’s a new way out. What if we could see through the noise without all the time & effort, without all the personal victimized legwork? Well, perhaps another technological innovation — artificial intelligence — can save the day.
The Future of News: Outsourced Attention
Enter: AI. The hopeful part of the human condition is that no matter how many sticky situations we put ourselves into, we’re usually able to innovate our way to a better place. Now, we are sitting at the vanguard of a new technological revolution, one where we may have invented a stand-in for our precious time & attention.
Perhaps we can’t change the incentives of ad-based news sites very easily. But thanks to advances in natural language processing and AI, it seems highly probable that we can train a system to do the legwork of aggregating & identifying high-value information for us — to outsource the discovery process and cut through the noise. At Babbl, we’re working on exactly that, and below we’ll outline how it can be done:

Introducing Uptrends: AI-based stock market news monitoring
Babbl is a startup building news & social media monitoring tools to take the legwork out of finding news insights. Using AI natural language processing models, we’re able to parse automatically parse through all news about a particular stock, sector, topic, or the market as a whole, and identify which insights carry the most value — the idea here is to exponentially improve the attention bandwidth of folks who use the news to make investment decisions. At a high level, Babbl’s model follows a four-step process to extract valuable information from news en masse:
1 Aggregating news content
It starts by scraping the web to gather content from a broad range of sources in real-time: accredited publishers like Yahoo Finance, CNBC, social media sites like Twitter, Reddit, StockTwits, and blog sites like Substack or SeekingAlpha. We parse thousands of sources and gather millions of articles per year.
2. Cleaning & filtering
From there, we process each sentence to identify subjects (ex: Apple stock) and topics (ex: earnings) — this involves training large language models that encode text into representations that a computer can understand. With subjects & topics identified, we can then group the mass of sentences into those about Apple, and filter out any snippets that are low-quality or irrelevant (ex: if you’re trying to find insight about Apple stock, you probably care more about sentences pertaining to the company’s financial performance, and less about sentences about how to jailbreak your iPhone).
3. Identifying valuable features
Third, and perhaps most importantly, once we’ve identified a core set of sentences (or strings of sentences) that are relevant to a stock’s (ie. Apple’s) stock performance, we can then identify relevant features about the opinion being expressed. We call this the sentiment. Sentiment features include mood (ie. the tone being expressed), tense (the time direction being referred to; past, present, or future), and other things like confidence or uncertainty. This process involves searching for keywords or grammatical patterns that are indicative of mood, tense, or confidence. Ultimately, this enables us to give each sentence a score for how “bullish” or “bearish” it is with respect to a given subject like Apple.
4. Synthesis of insight
Finally, with a clean set of scored, relevant sentences about a given subject, we can then measure the overall connotation being expressed en masse about said subject. This basically entails taking a weighted sum of all the sentences (where weight represents the importance of a given sentence pertaining to a subject) to end up at a singular “bullish” or “bearish”. For interpretability, we normalize the score of a given subject on a scale from -100 (highly bearish) to +100 (highly bullish). From here, we can track the sentiment of a subject over time, across various sources, and relative to other subjects.
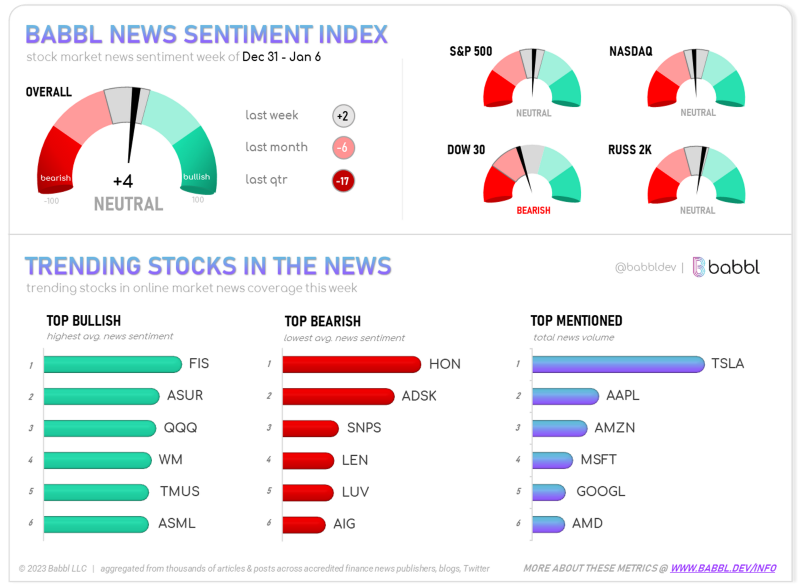
When Actionable Information Grows on Trees
Now imagine, when discovery & synthesis is no longer a solely manual process, what are the possibilities? When we can automatically detect & quantify the most important events, and associate their impact on tangible stock market outcomes. Can we see into the future? To check out our process and have a closer look at what we’re building at Uptrends, visit www.uptrends.ai to see for yourself.